Nicolas Perez
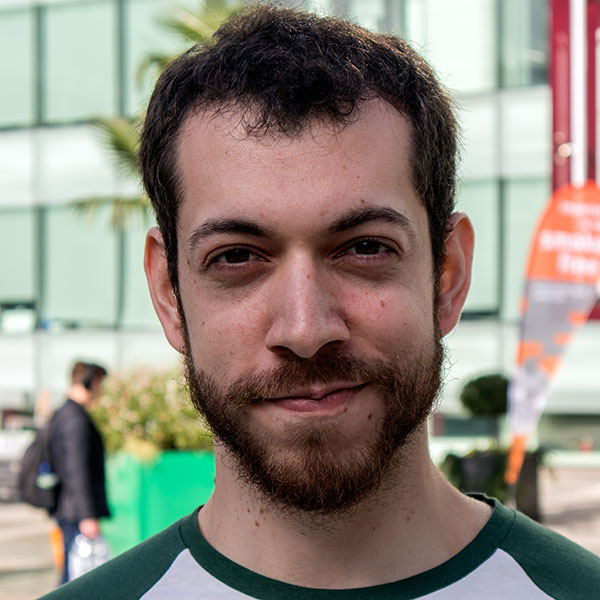
-
- Lab member: 2018-2023
-
PhD thesis
Robust and efficient training on deep spiking neural networks
Nicolas Perez was a PhD student working on understanding how spiking neural networks can use heterogeneous neuron properties to carry out multiresolution processing of sensory data. After his PhD he moved to DeepMind.
Videos
-
Understanding the role of neural heterogeneity in learningTalk / 2021
Talk on neural heterogeneity by Nicolas Perez. -
Neural heterogeneity promotes robust learningTalk / 2021
Talk on neural heterogeneity by Dan Goodman.
Publications
Note that only publications as part of the Neural Reckoning group are included here (see external publications below for full list).
2023
-
Perez N (2023)
Robust and efficient training on deep spiking neural networks.
PhD thesis, Imperial College London
2022
-
Goodman D, Fiers T, Gao R, Ghosh M, Perez N (2022)
Spiking Neural Network Models in Neuroscience - Cosyne Tutorial 2022.
Zenodo
2021
-
Perez-Nieves N, Goodman DFM (2021)
Sparse spiking gradient descent.
Advances in Neural Information Processing Systems -
Perez-Nieves N, Leung VCH, Dragotti PL, Goodman DFM (2021)
Neural heterogeneity promotes robust learning.
Nature Communications
2019
-
Perez-Nieves N, Leung VCH, Dragotti PL, Goodman DFM (2019)
Advantages of heterogeneity of parameters in spiking neural network training.
Cognitive Computational Neuroscience - + 1 conference paper
External publications
This is a short preview of the publications from other sources (ORCID, Semantic Scholar). Note that publications from work done outside the Neural Reckoning group are included in this list.
2025
2023
- Adaptive Agent Team, et al. (2023)
Human-Timescale Adaptation in an Open-Ended Task Space
International Conference on Machine Learning
2021
- D. Mguni, et al. (2021)
Learning to Shape Rewards Using a Game of Two Partners
AAAI Conference on Artificial Intelligence - Nicolas Perez Nieves, Dan F. M. Goodman (2021)
Sparse Spiking Gradient Descent
Neural Information Processing Systems - D. Mguni, et al. (2021)
LIGS: Learnable Intrinsic-Reward Generation Selection for Multi-Agent Learning
International Conference on Learning Representations - Nicolas Perez Nieves, Yaodong Yang, Oliver Slumbers, D. Mguni, Jun Wang (2021)
Modelling Behavioural Diversity for Learning in Open-Ended Games
International Conference on Machine Learning
2020
- Ming Zhou, et al. (2020)
SMARTS: Scalable Multi-Agent Reinforcement Learning Training School for Autonomous Driving
arXiv.org